ARL Weekly News – September 23, 2022.
Recent Events |
Emissions measurements in Baltimore’s Curtis Bay presented to Maryland Department of the Environment
On Friday September 16, Phil Stratton presented a preliminary analysis of mobile measurements he and Xinrong Ren made between March and May of this year. The presentation was given to the Maryland Department of the Environment and any general public in attendance at their public quarterly meeting with collaborators from the University of Maryland and Johns Hopkins University. The talk focused on the emission and source characteristics of methane, ethane, black carbon, and carbon monoxide in the Curtis Bay area in Baltimore and illustrated the complexity of making observations in a diverse urban environment. Presenting this preliminary work shows ARL’s contribution to creating an independent, transparent evaluation of GHG emissions and the changes in emissions at various scales.
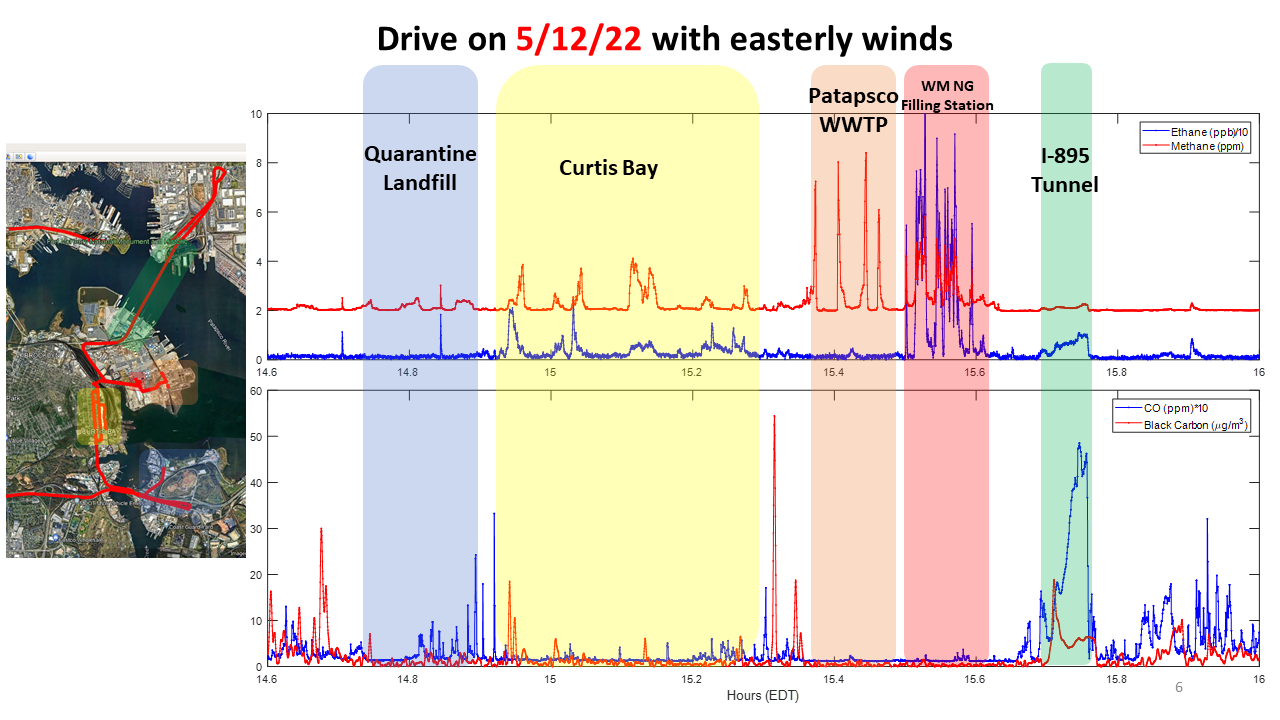
Emissions measurements around Curtis Bay on May 12, with easterly wind direction.
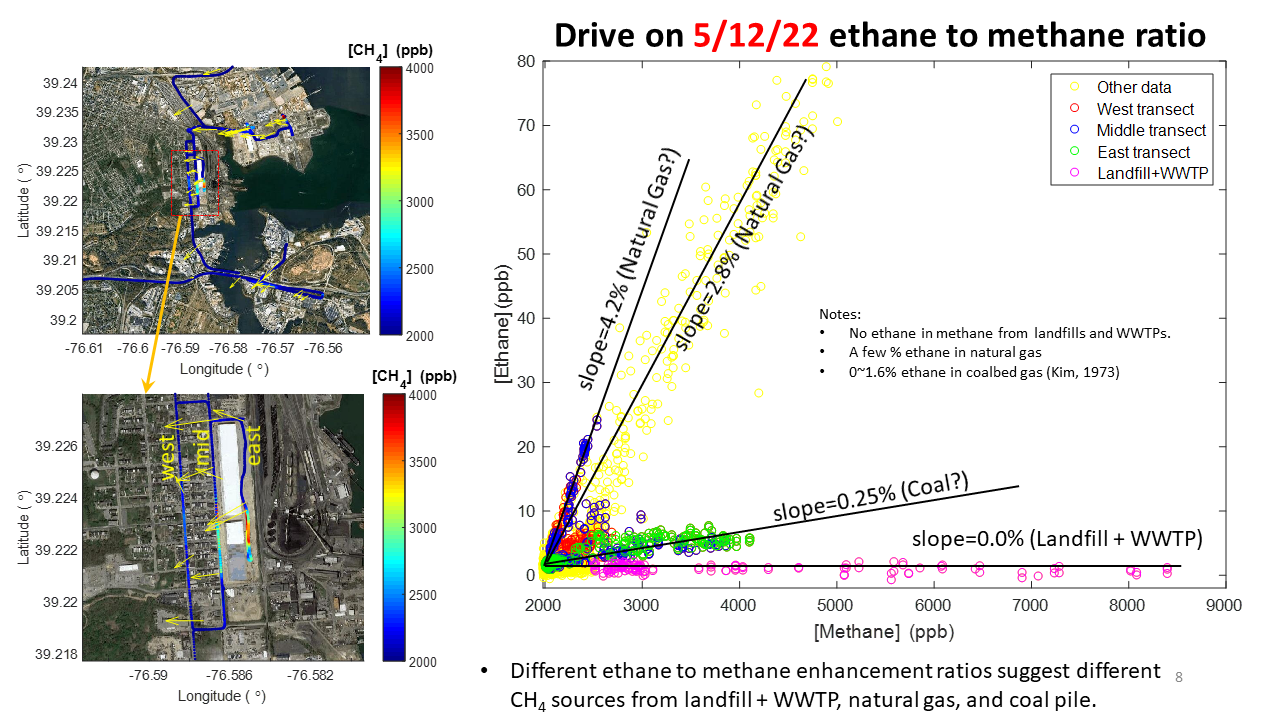
Drive on May 12 demonstrating calculated ethane to methane ratios.
HYSPLIT models emissions from a Tesla Megapack battery fire California
A Tesla Megapack caught fire at a PG&E energy storage facility in Monterey, California on Tuesday, September 20. The 182.5-megawatt (MW) Tesla Megapack system, known as the Elkhorn Battery at Moss Landing, was installed in April this year. Richard Stedman, an air pollution control officer for the Monterey Bay Air Resources District (MBARD) said in general lithium ion battery fires can emit toxic constituents like hydrochloric and hydrofluoric acid.
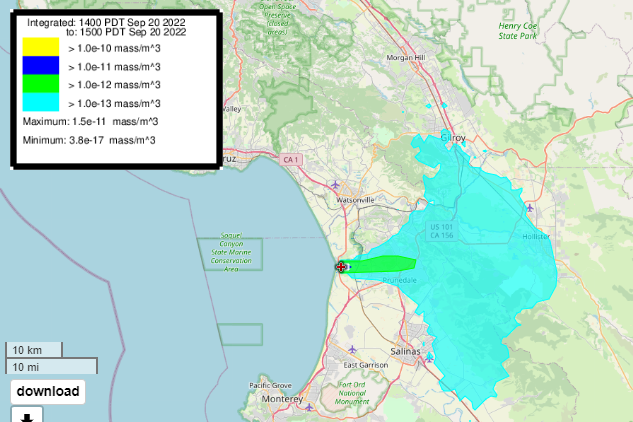
Moss Landing HYSPLIT plume calculations for September 20, 2022.
ICARS Symposium
Nebila Lichiheb will presented a paper on: Improving the prediction of hazardous material dispersion in an urban environment to the IC Academic Research Symposium on Wednesday September the 28th, 2022.
Published: Machine Learning for Improving Surface-Layer-Flux Estimates
McCandless, T., Gagne, D.J., Kosović, B., Haupt, S.E., Yang, B., Becker, C., & Schreck, J., Machine Learning for Improving Surface-Layer-Flux Estimates. Boundary-Layer Meteorol (2022). https://doi.org/10.1007/s10546-022-00727-4
Flows in the atmospheric boundary layer are turbulent, characterized by a large Reynolds number, the existence of a roughness sublayer and the absence of a well-defined viscous layer. Exchanges with the surface are therefore dominated by turbulent fluxes. In numerical models for atmospheric flows, turbulent fluxes must be specified at the surface; however, surface fluxes are not known a priori and therefore must be parametrized. Atmospheric flow models, including global circulation, limited area models, and large-eddy simulation, employ Monin–Obukhov similarity theory (MOST) to parametrize surface fluxes. The MOST approach is a semi-empirical formulation that accounts for atmospheric stability effects through universal stability functions. The stability functions are determined based on limited observations using simple regression as a function of the non-dimensional stability parameter representing a ratio of distance from the surface and the Obukhov length scale (Obukhov in Trudy Inst Theor Geofiz AN SSSR 1:95–115, 1946), z/Lz/L. However, simple regression cannot capture the relationship between governing parameters and surface-layer structure under the wide range of conditions to which MOST is commonly applied. We therefore develop, train, and test two machine-learning models, an artificial neural network (ANN) and random forest (RF), to estimate surface fluxes of momentum, sensible heat, and moisture based on surface and near-surface observations. To train and test these machine-learning algorithms, we use several years of observations from the Cabauw mast in the Netherlands and from the National Oceanic and Atmospheric Administration’s Field Research Division tower in Idaho. The RF and ANN models outperform MOST. Even when we train the RF and ANN on one set of data and apply them to the second set, they provide more accurate estimates of all of the fluxes compared to MOST. Estimates of sensible heat and moisture fluxes are significantly improved, and model interpretability techniques highlight the logical physical relationships we expect in surface-layer processes.